Special Session:
AI in Healthcare: From Model Development to Deployment – Challenges and Opportunities
Artificial Intelligence (AI) has revolutionized the healthcare industry by enabling faster diagnoses, personalized treatment plans, and efficient health data management. However, despite its potential, AI deployment in healthcare faces several critical challenges. High computational costs, privacy concerns, lack of transparency, and difficulties in model generalization hinder its widespread adoption in real-world clinical settings. Many AI models require large-scale, centralized datasets for training, which raises ethical and legal concerns regarding patient data privacy and security. Techniques such as federated learning and split learning offer promising solutions by allowing decentralized AI training while preserving data confidentiality. Moreover, deep neural networks are often computationally expensive, making them impractical for deployment on resource-constrained devices, such as portable medical sensors, wearable devices, and edge computing systems. This highlights the need for model compression techniques (pruning, quantization, knowledge distillation) to reduce complexity without sacrificing accuracy. Additionally, explainability and robustness remain major barriers to AI adoption in healthcare. Clinicians require AI models to be interpretable, reliable, and resistant to adversarial attacks before they can be trusted in critical decision-making scenarios. Methods like uncertainty quantification and adversarial defense strategies ensure AI models provide trustworthy insights.
Topics of Interest
Given these challenges, this special session will focus on advancing AI methodologies for secure, efficient, and explainable healthcare applications, addressing the technical hurdles that must be overcome for AI to become truly scalable, privacy-preserving, and clinically reliable.
We invite contributors involved both in research institutions and industry to submit their proposed works to address key topics to be covered in this session, but not limited to:
- Explainability and Trust in AI: Ensuring transparency and robustness in AI-driven decision-making
- Privacy-Preserving AI: Federated learning, split learning, and secure data sharing techniques
- Model Efficiency and Complexity Reduction: Compression methods (pruning, quantization, knowledge distillation)
- State-of-Art AI Systems in Healthcare: Robust, scalable, decentralized AI solutions for real-time medical applications
Acknowledgment
All these research topics fall within the objectives of the joint Turkish-Tunisian Project: Reliable and Explainable AI Approaches for Medical Image Understanding, which has been accepted for the Call for Proposal 2024.
Prospective authors of papers are invited to submit a paper (typically 4-6 pages in standard IEEE two-column A4 format) via EDAS platform by suggesting the related special session: link. The paper should contain a complete description of the proposed contribution along with results, suitably framed in the related state of the art. Each paper will be reviewed in terms of relevance with respect to the scope of the event, originality and quality of the technical content, overall organization and writing style.
The IEEE Style Manual and Conference Paper templates in various formats are available through the following links:
http://www.ieee.org/conferences_events/conferences/publishing/templates.html
Deadlines
- Papers Submission Deadlines – April 30, 2025
- Notification of Papers Acceptance – May 15, 2025
- Final Paper Camera-ready Submission – May 31, 2025
- Early Bird Registration Deadline – May 31, 2025
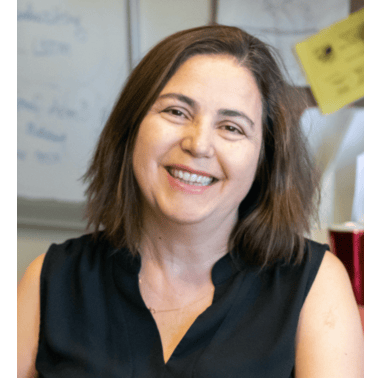
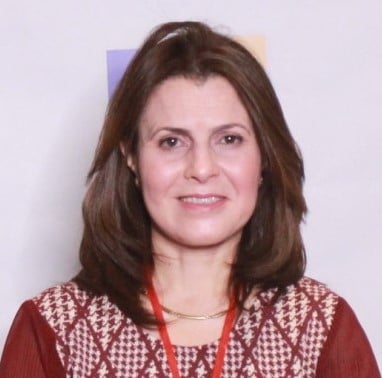
- Ghazala Hcini, University of Sfax
- Jihene Tmamna, University of Sfax